Why Big Data Needs Thick Data
Originally published on May 13, 2013 for Ethnography Matters, I’m republishing the post for the launch of the new Ethnography Matters Medium channel. I’ve updated the article with a case study from my time at Nokia where I witnessed their over-dependence on quantitative data. I’m continuously blown away by how much my original post in 2013 sparked a discussion about the integration of Thick Data and Big Data. Since then, I have talked about this topic with EPIC conference, Republica and Strata. I also gave a TED talk targeted for business leaders. Just last year, Word Spy created an entry of Thick Data, referencing my original post as the resurgence of the term. My goal is to create more opportunities to feature people who are doing this kind of integrative work inside organizations. Please reach out if this kind of work is up your alley. You can start by joining a community of people who are at the forefront of this work at Ethnography Hangout Slack’s #datatalk channel. Thank you to IDEO’s Elyssa He for translating this article into Chinese, 大数据离不开 “厚数据”, published on 36kr and Paz Bernaldo for translating this into Spanish.
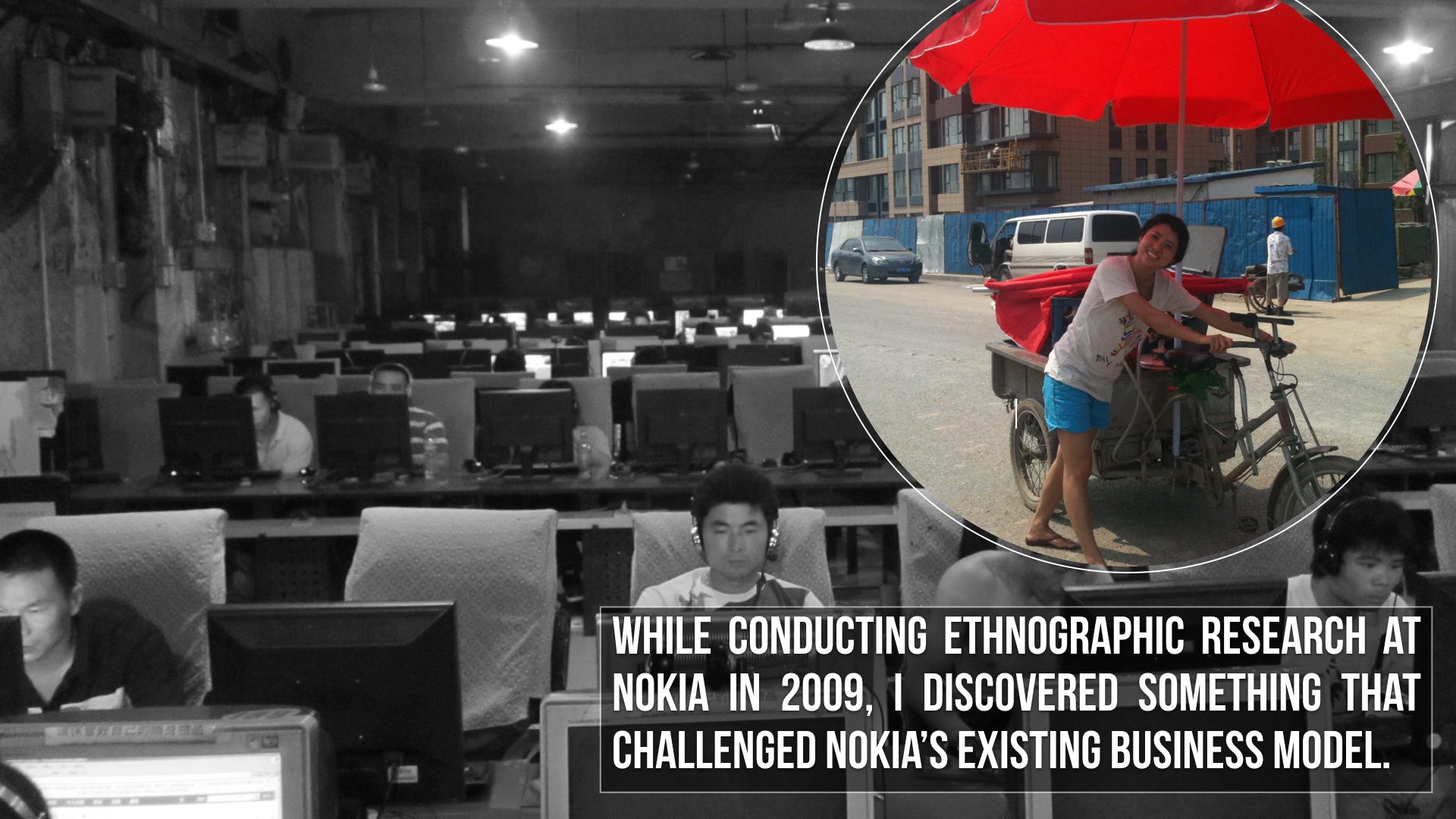
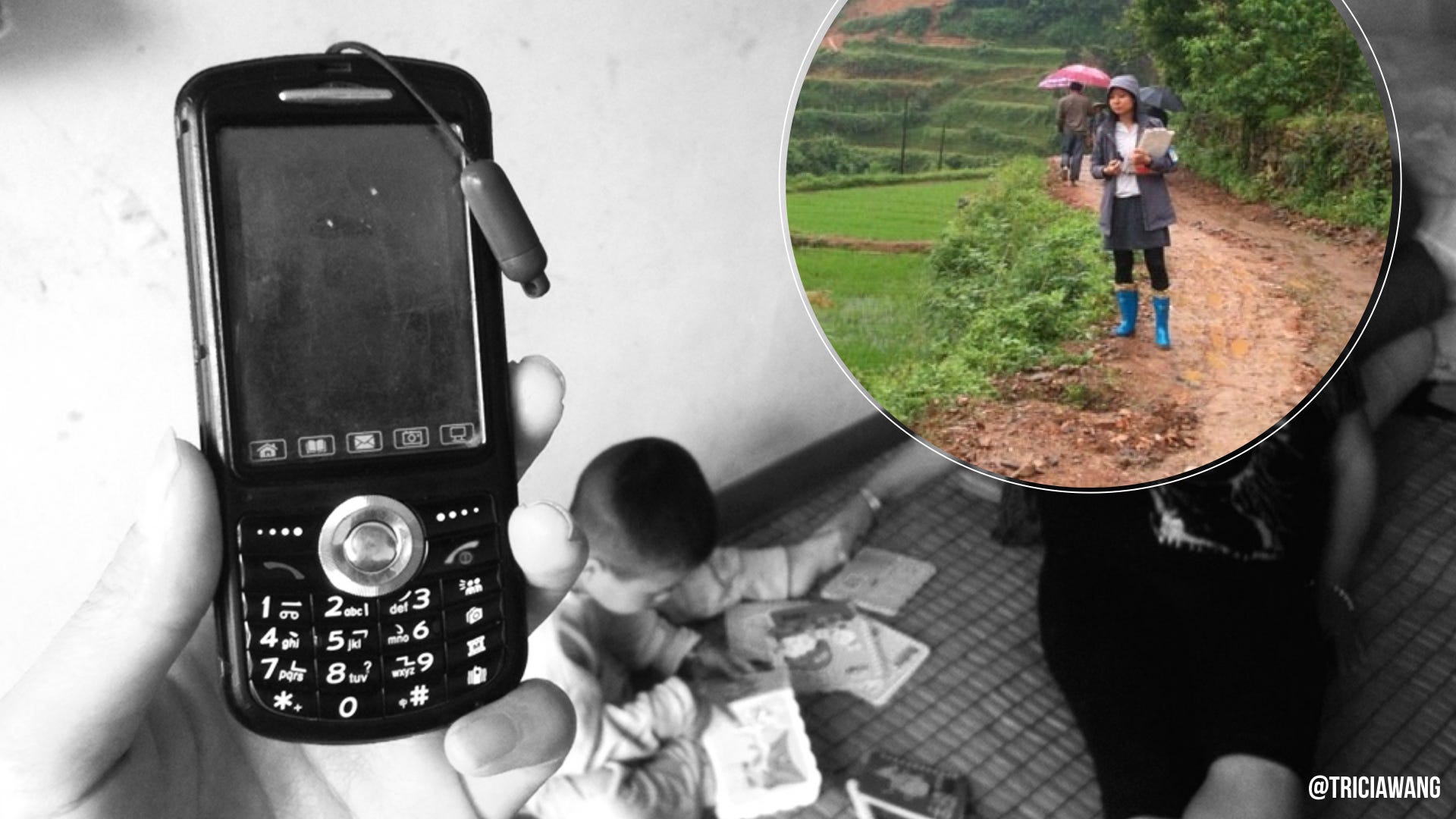
When I was researching at Nokia in 2009, which at the time was the world’s largest cellphone company in emerging, I discovered something that I believed challenged their entire business model. After years of conducting ethnographic work in China from living with migrants to working as a street vendor and living in internet cafés, I saw lots of indicators that led me to conclude that low-income consumers were ready to pay for more expensive smartphones.
I concluded that Nokia needed to replace their current product development strategy from making expensive smartphones for elite users to affordable smartphones for low-income users. I reported my findings and recommendations to headquarters. But Nokia did not know what to do with my findings. They said my sample size of 100 was weak and small compared to their sample size of several million data points. In addition, they said that there weren’t any signs of my insights in their existing datasets.
In response, I told them that it made sense that they haven’t seen any of my data show up in their quantitative datasets because their notion of demand was a fixed quantitative model that didn’t map to how demand worked as a cultural model in China. What is measurable isn’t the same as what is valuable.
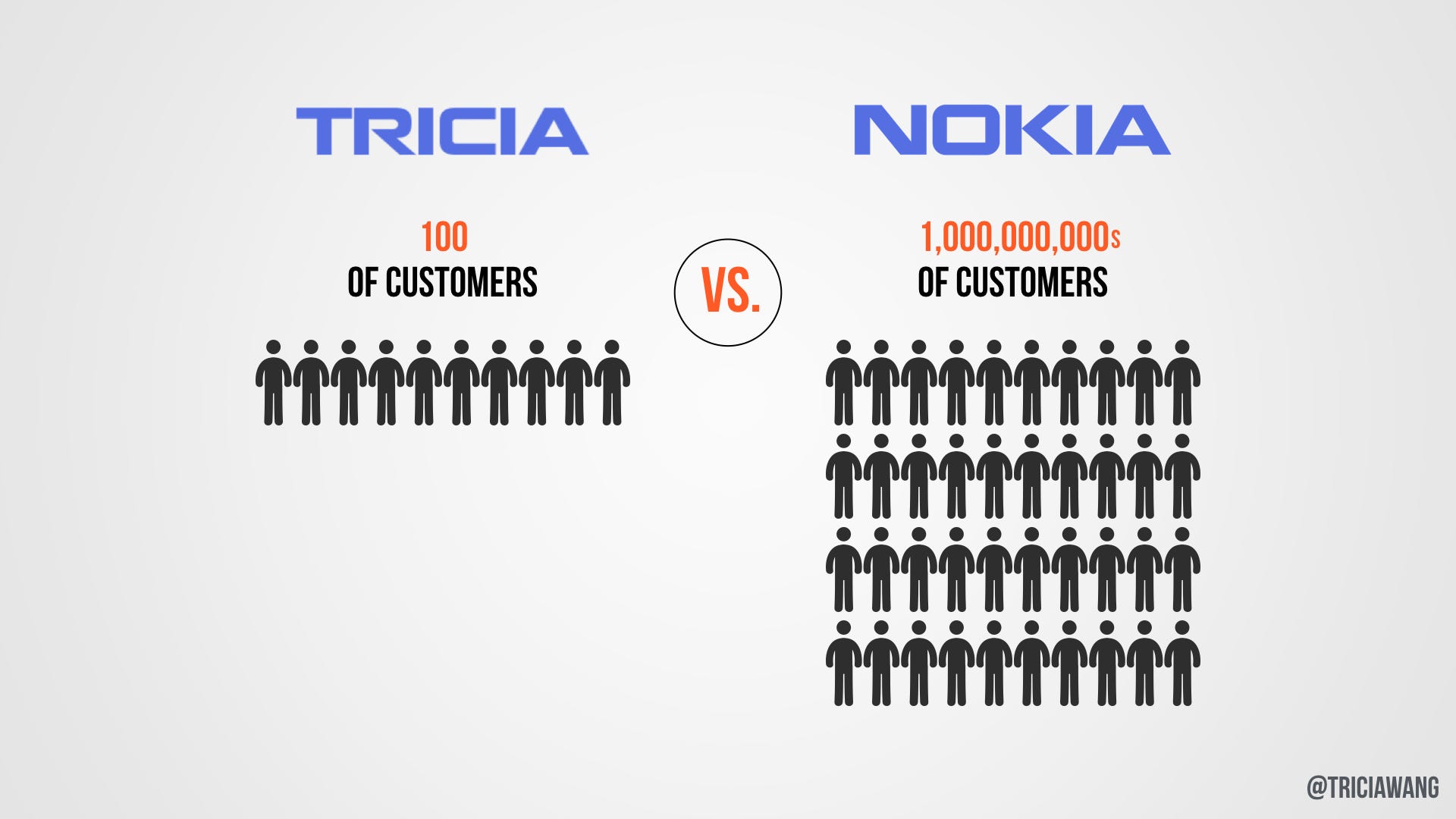
By now, we all know what happened to Nokia. Microsoft bought them in 2013 and it only has three percent of the global smartphone market. There are many reasons for Nokia’s downfall, but one of the biggest reasons that I witnessed in person was that the company over-relied on numbers. They put a higher value on quantitative data, they didn’t know how to handle data that wasn’t easily measurable, and that didn’t show up in existing reports. What could’ve been their competitive intelligence ended up being their eventual downfall.
Since my time at Nokia, I’ve been very perplexed by why organizations value quantitative more than qualitative data. With the rise of Big Data, I’ve seen this process intensify with organizations investing in more big data technology while decreasing budgets for human-centered research. I’m deeply concerned about the future of qualitative, ethnographic work in the Era of Big Data.
Ethnographic work has a serious perception problem in a data-driven world. While I’ve always integrated statistical analysis into my qualitative work in academia, I encountered a lot of doubt on the value of ethnographically derived data when I started working primarily with corporations. I started to hear echoes of what Nokia leadership said about my small dataset, that ethnographic data is “small” “petite” “puny.”. What are ethnographers to do when our research is seen as insignificant or invaluable? How can our kind of research be seen as an equally important to algorithmically processed data? To solve this perception problem, ethnographers need a 10 second elevator pitch to a room of data scientists.
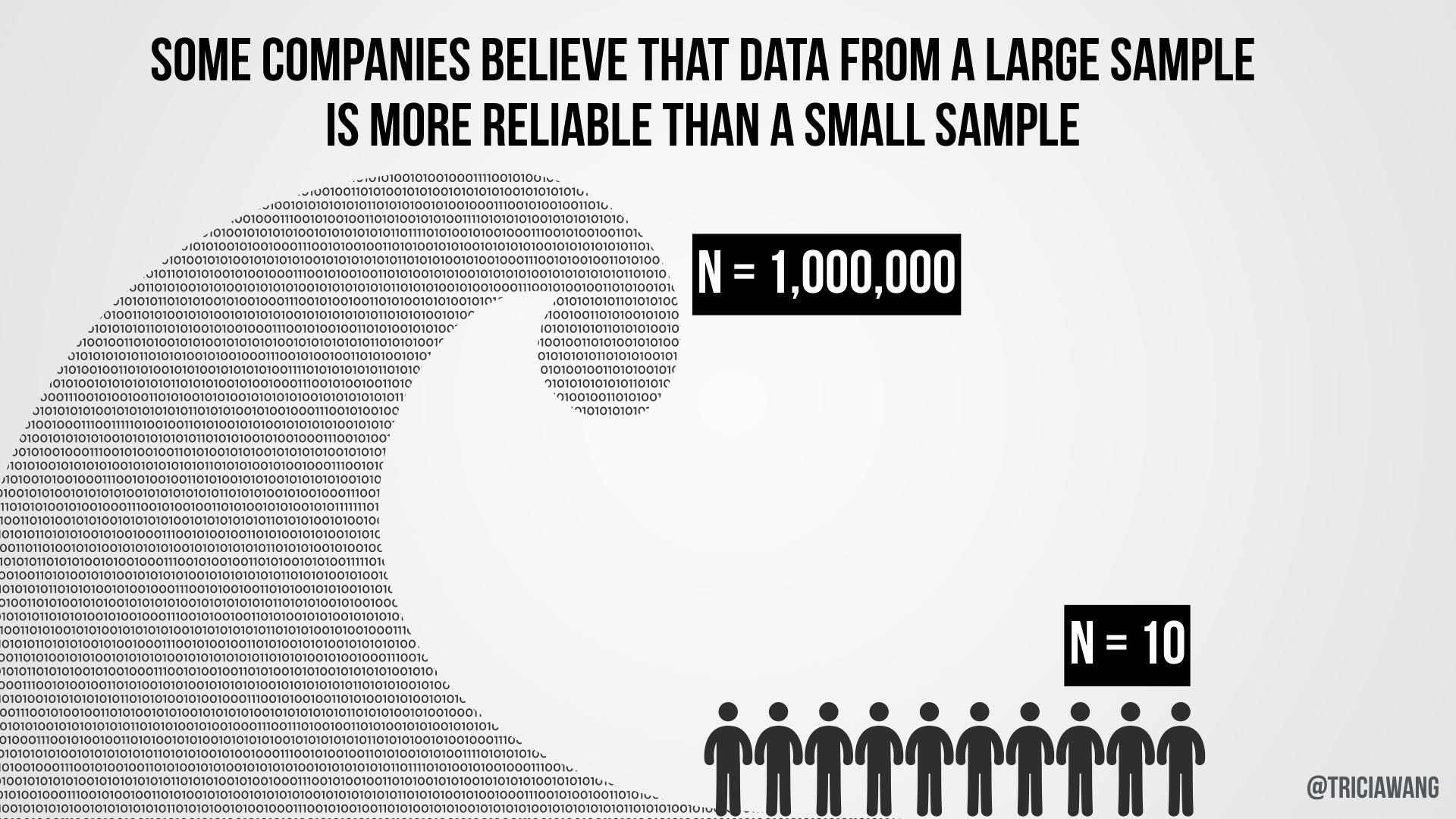
Lacking the conceptual words to quickly position the value of ethnographic work in the context of Big Data, I have begun, over the last year, to employ the term Thick Data (with a nod to Clifford Geertz!) to advocate for integrative approaches to research. Thick Data is data brought to light using qualitative, ethnographic research methods that uncover people’s emotions, stories, and models of their world. It’s the sticky stuff that’s difficult to quantify. It comes to us in the form of a small sample size and in return we get an incredible depth of meanings and stories. Thick Data is the opposite of Big Data, which is quantitative data at a large scale that involves new technologies around capturing, storing, and analyzing. For Big Data to be analyzable, it must use normalizing, standardizing, defining, clustering, all processes that strips the the data set of context, meaning, and stories. Thick Data can rescue Big Data from the context-loss that comes with the processes of making it usable.
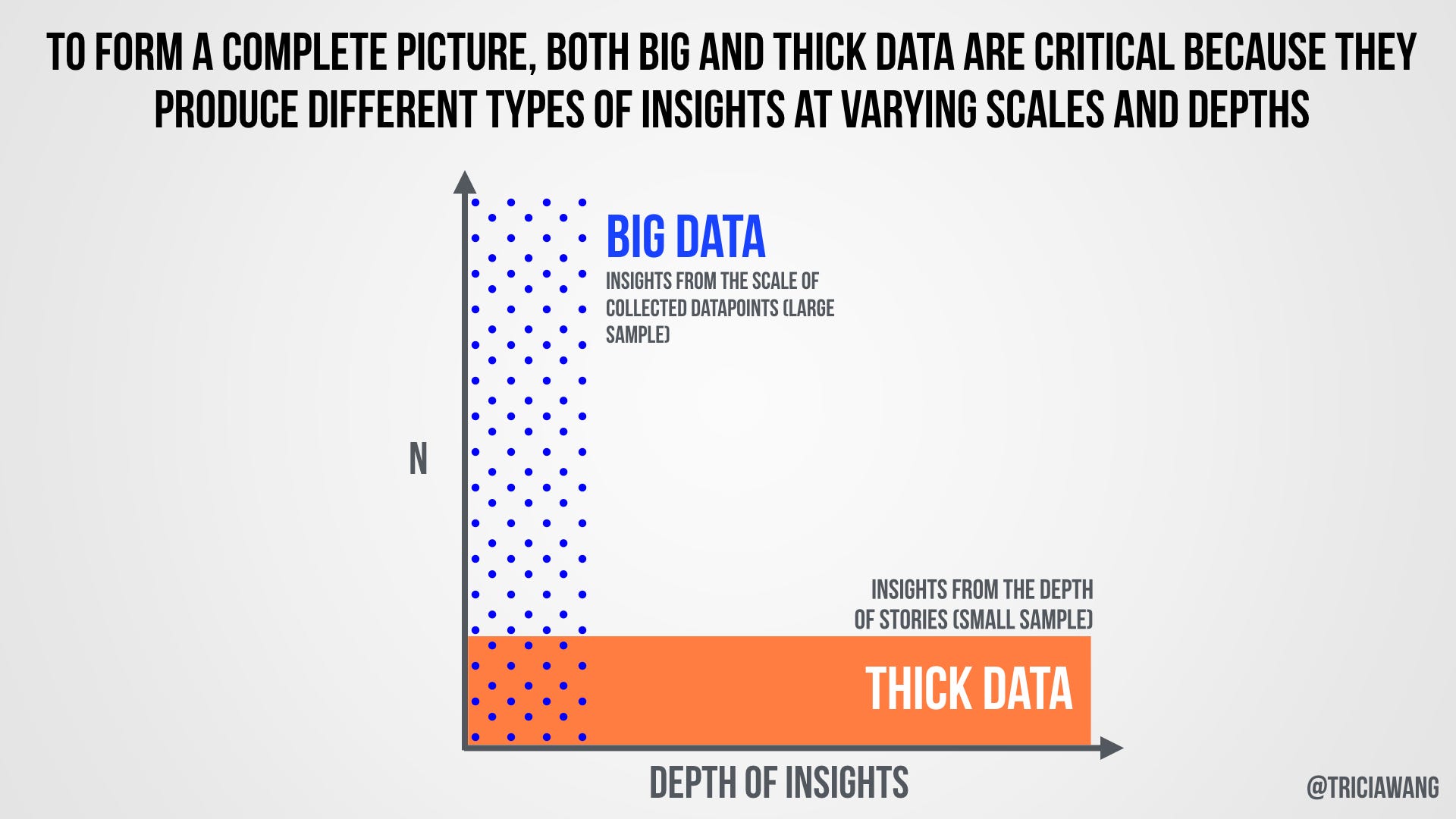
Integrating big data and thick data provides organizations a more complete complete context of any given situation. For businesses to form a complete picture, they need both big and thick data because each of them produce different types of insights at varying scales and depths. Big Data requires a humongous N to uncover patterns at a large scale while Thick Data requires a small N to see human-centered patterns in depth. Both types of Thick Data relies on human learning, while Big Data relies on machine learning. Thick Data reveals the social context of connections between data points while Big Data reveals insights with a particular range of quantified data points. Thick Data techniques accepts irreducible complexity, while Big Data techniques isolates variables to identify patterns. Thick Data loses scale while Big Data loses resolution.
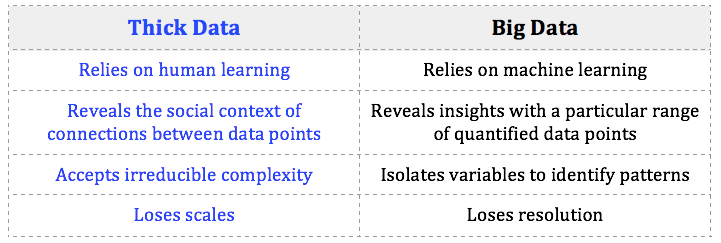
CAUTION AHEAD
As the concept of “Big Data” has become mainstream, many practitioners and experts have cautioned organizations to adopt Big Data in a balanced way. Many qualitative researchers from Genevieve Bell to Kate Crawford and danah boyd have written essays on the limitations of Big Data from the perspective of Big Data as a person, algorithmic illusion, data fundamentalism, and privacy concerns respectively. Journalists have also added to the conversation. Caribou Honig defends small data, Gary Marcus cautions about the limitations of inferring correlations, Samuel Arbesman calls for us to move on to long data. Jenna Burrell has produced a guide for ethnographers to understand big data.
Inside organizations Big Data can be dangerous. Steven Maxwell points out that “People are getting caught up on the quantity side of the equation rather than the quality of the business insights that analytics can unearth.” More numbers do not necessarily produce more insights.
Another problem is that Big Data tends to place a huge value on quantitative results, while devaluing the importance of qualitative results. This leads to the dangerous idea that statistically normalized and standardized data is more useful and objective than qualitative data, reinforcing the notion that qualitative data is small data.
These two problems, in combination, reinforce and empower decades of corporate management decision-making based on quantitative data alone. Corporate management consultants have long been working with quantitative data to create more efficient and profitable companies.
Without a counterbalance the risk in a Big Data world is that organizations and individuals start making decisions and optimizing performance for metrics — metrics that are derived from algorithms. And in this whole optimization process, people, stories, actual experiences, are all but forgotten. The danger, writes Clive Thompson, is that “by taking human decision-making out of the equation, we’re slowly stripping away deliberation — moments where we reflect on the morality of our actions.”
INSPIRATION and EMOTION
Big Data produces so much information that it needs something more to bridge and/or reveal knowledge gaps. That’s why ethnographic work holds such enormous value in the era of Big Data. Below, I explore some the ways that organizations are integrating Thick Data.
Thick Data is the best method for mapping unknown territory. When organizations want to know what they do not already know, they need Thick Data because it gives something that Big Data explicitly does not — inspiration. The act of collecting and analyzing stories produces insights.
Stories can inspire organizations to figure out different ways to get to the destination — the insight. If you were going to drive, Thick Data is going to inspire you to teleport. Thick Data often reveals the unexpected. It will frustrate. It will surprise. But no matter what, it will inspire. Innovation needs to be in the company of imagination.
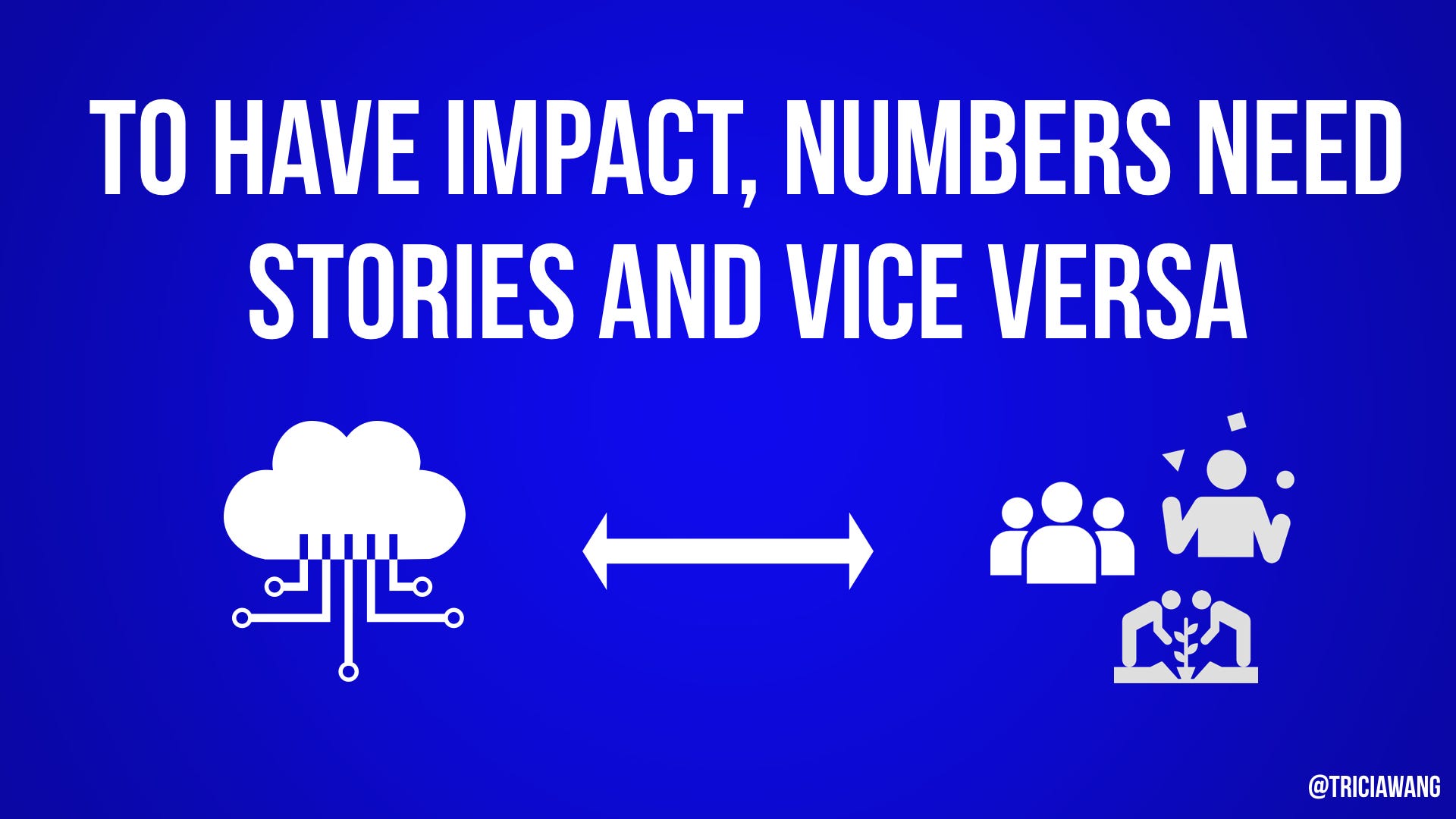
When organizations want to build stronger ties with stakeholders, they need stories. Stories contain emotions, something that no scrubbed and normalized dataset can ever deliver. Numbers alone do not respond to the emotions of everyday life: trust, vulnerability, fear, greed, lust, security, love, and intimacy. It’s hard to algorithmically represent the strength of an individual’s service/product affiliation and how the meaning of the affiliation changes over time. Thick Data approaches reach deep into people’s hearts. Ultimately, a relationship between a stakeholder and an organization/brand is emotional, not rational.
Some people are uncomfortable with the use of the term “stories” to describe ethnographic work. There’s a lot of confusion that stories are the equivalent to anecdotes. Market researchers question if it is a “fad.” Even in academia, many sociologists shun the use of “stories” because it makes their qualitative work appear less scientific. In my PhD Sociology program, professors often told us to use “cases” instead of “stories.”
There’s a big difference between anecdotes and stories, however. Anecdotes are casually gathered stories that are casually shared. Within a research context, stories are intentionally gathered and systematically sampled, shared, debriefed, and analyzed, which produces insights (analysis in academia). Great insights inspire design, strategy, and innovation.
NPR has a segment that illustrates the power of Thick Data, featuring Frans de Waal, a primatologist and biologist who just published “The Bonobo and the Atheist: In Search of Humanism Among the Primates”. Through his experiments, de Waal provides evidence to support his theory that a sense of fairness — the groundwork for morality — is not unique to humans. In his talks, de Waal shows a video of two Capuchin monkeys receiving different rewards for performing the same action. The monkey that gets a cucumber becomes very upset when she sees the monkey next to her given a grape as a reward for performing a similar task. In the monkey world, grapes are crack and cucumbers are stale bread.
In his research statement, de Waal makes a captivating case for the principles of Thick Data:
“I show that video often, because if I show the data, which are graphs and stuff like that, people are not really convinced, if you show the emotional reaction, the amount of emotion that goes in there, then people are convinced.”
As de Waal makes clear, sometimes the quantitative data alone will not make a compelling argument. Even scientists need stories to make their point.
OPPORTUNITIES
While using Big Data in isolation can be problematic, it is definitely critical to continue exploring how Big Data and Thick Data can complement each other. This is a great opportunity for qualitative researchers to position our work in the context of Big Data’s quantitative results. Companies like Claro Partners are even reframing the way we ask questions about Big Data. In their Personal Data Economy research, instead of asking what Big Data tells us about human behavior, they asked what human behavior tells us about the role of Big Data in everyday life. They created a toolkit for clients that helps them shift their “perspective from a data-centric one to a human-centred one.”
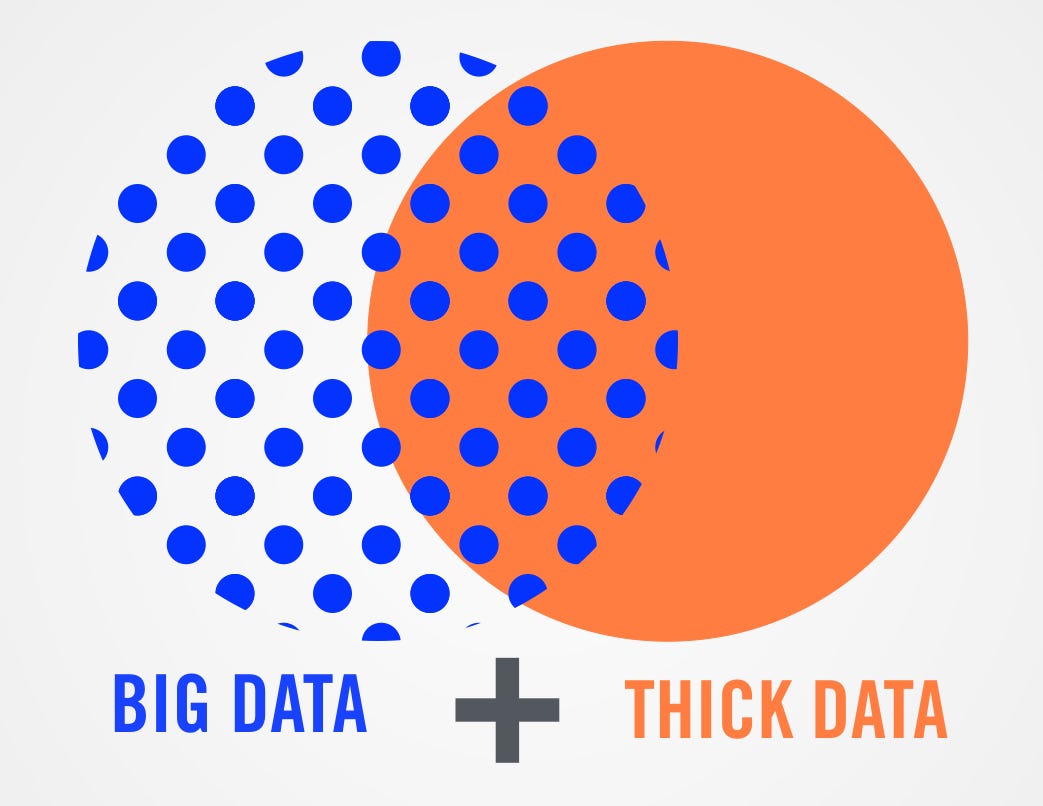
Here are some areas where I see opportunity for collaboration between the two methods within organizations (this is not meant to an exhaustive or comprehensive list).
Health Care — As individuals have become more empowered to monitor their own health, Quantified Self values are going mainstream. Health providers will have increased access to collectively sourced, anonymized data. Projects such as Asthma Files provide a glimpse into the future of Thick and Big Data partnerships to solve global health problems.
Repurposed anonymized data from mobile operators — Mobile companies around the world are starting to repackage and sell their customer data. Marketers are not the only buyers. City planners who want better location-based data to understand transportation are using Air Sage’s cellular network data. To protect user privacy, the data is either anonymized or deliberately scrubbed of personal communication. And yet in the absence of key personal details, the data loses key contextual information. Without Thick Data, it will be difficult for organizations to understand the personal and social context of data that has been scrubbed of personal information.
Social Network Analysis — Social media produces droves of data that can enrich social network analysis. Research scientists such as Hilary Mason, Gilad Lotan, Duncan Watts, and Ethan Zuckerman (and his lab at MIT Media Lab) are exploring how information spreads on social networks and, at the same time, are creating more questions that can only be answered by using Thick Data methods. As more companies make use of social media metrics, organizations have to be careful not to mistakenly believe that data alone will reveal “influencers.” An example of misinterpreting a signal from Big Data network analysis is the media’s write up of Cesar Hildalgo’s work, suggesting that Wikipedia could serve as a proxy for culture. (Read Heather Ford’s correction.)
Brand Strategy and Generating Insight — Companies have long relied on market analysis to dictate corporate strategy and insight generation. They are now turning to a more user-centered approach that relies on Thick Data. Fast Company’s recent feature of Jcrew makes clear that where Big Data driven management consultants failed, the heroes that led a brand’s turnaround were employees who understood what consumers wanted. One employee, Jenna Lyons was given the opportunity to implement iterative, experimental, and real-time testing of products with consumers. Her approach resonated with consumers, transforming Jcrew into a cult brand and tripling its revenues.
Product/Service Design — Algorithms alone do not solve problems, and yet many organizations rely on them for product and service design. Xerox uses Big Data to solve problems for the government, but they also use ethnographic methods alongside analytics. Ellen Issacs, a Xerox PARC ethnographer speaks to the importance of Thick Data in design: “[e]ven when you have a clear concept for a technology, you still need to design it so that it’s consistent with the way people think about their activities . . . you have to watch them doing what they do.”
Implementing Organizational Strategy — Thick Data can be used as a counterbalance to Big Data to mitigate the disruptiveness of planned organizational change. Quantitative data may suggest that a change is needed, but disruption inside organizations can be costly. When organizational charts are rearranged, job descriptions are rewritten, job functions shift, and measures of success are reframed — the changes can cause a costly disruption that may not show up in the Big Data plan. Organizations need Thick Data experts to work alongside business leaders to understand the impact and context of changes to from a cultural perspective to determine which changes are advisable and how to navigate the process. Grant McCracken calls this the Chief Cultural Officer, the “corporation’s eyes and ears, allowing it to detect coming changes, even when they exist only as the weakest of signals.” The CCO is the go to Thick Data person, responsible for collecting, telling, and circulating stories to keep an organization inspired and agile. Roger Magoulas, who coined the term Big Data, emphasizes the need for stories: “stories tend to spread quickly, helping spread the lessons from the analysis throughout an organization.”
FUTURE
The integration of Thick Data raises new questions for organizations.
- How do we report Thick Data up? Stories are effective, but stories require time, resource, and communication skills.
- What are the indicators for successful Thick Data research?
- How do we train teams in integrative Big Data and Thick Data approaches?
There is greater demand for ethnographers as suppliers/providers than as employees inside organizations. There are not enough ethnographers working inside companies to internalize ethnographic research and to explore different ways to extend the insights of Big and Thick Data.
Even though we have a lot of questions to answer about Thick Data, it’s important to keep in mind that this is the time for ethnographic work to really shine. We’re in a great position to show the value-add we bring to a mixed-method project. Producing “thick descriptions” (a term used by Clifford Geertz to describe ethnographic methodology) of a social context compliments Big Data findings. People and organizations pioneering Big Data and Thick Data projects, such as Fabien Giradin from the Near Future Laboratory or Wendy Hsu, are giving us glimpses into this world.
It’s also important to remember that there is a lot of progress to be made on Big Data. According to a Gartner study of companies who had invested in Big Data capabilities, only 8% of were doing anything significant with their Big Data. The rest were only using Big Data for incremental advances. This means that a lot of companies are talking and investing in Big Data, but they aren’t doing anything transformational with it.
For enterprise and institutions to realize the full potential of Big Data, I believe they they need Thick Data. And that is why ethnographers are more needed now than ever. We play a critical role in keeping organizations human-centered in the era of Big Data.
_________________
Since writing this updated article in 2015, I gave a talk at TEDx with a business audience in mind for why every organization needs to prioritize Thick Data and the integration of it with Big Data. If you’re struggling to get alignment for the importance for Thick Data, share this video with your leadership and management teams.
Comments
Post a Comment
Please share your valuable comments and thoughts on this article. Thanks!